Gait disorders are common in society and occur in over 20% of geriatric patients (International Parkinson and Movement Disorder Society, 2019). The consequences of gait disorders can be serious. In addition to a slow and unsteady gait, falls can also occur more frequently. Due to the fear of falling, the affected persons increasingly stay at home and feel restricted in their daily life, so that the quality of life suffers greatly.
Step length for quantification of gait disorders
Most gait disorders can be diagnosed and classified by quantifying the gait pattern. For the monitoring and assessment of gait disorders, various spatial parameters such as step length are often used. An example of a disease in which step length is an important indicator is Parkinson’s disease, where affected persons take slow, small, shuffling steps.
Inertial sensors as mobile gait analysis
Technical devices, among others, are used to make the diagnosis and to document the disability as well as changes in the period of time. So-called inertial sensor units, or IMUs for short, which consist of an acceleration sensor, a gyroscope and a magnetometer, are becoming increasingly popular. The main advantages of these sensors are their small size and the fact that the measurements are not bound to a specific location. Marin et al. (2015) describe their use in motion analysis as follows:
The use of inertial measurement units in biomechanics and sports biomechanics has been, is, and will be not only promising but also a breakthrough in motion analysis.
Step length estimation using inertial sensors
A large amount of scientific literature deals with the estimation of the step or stride length using inertial sensors. The existing methods can mainly be assigned to two approaches:
(1) (bio)mechanical models
The model-based approach defines analytical expressions for step length estimation based on the geometric relationships between dimensions, angles, and displacements of different body parts. This is possible due to the mechanical nature of the human body (Diez et al., 2018). Derived from this, model-based approaches use an (inverse) double pendulum model to model the gait phase (Hannik et al., 2016).
(2) Double-Integration
The most commonly used approach to estimate step length is based on the double integration method. For the gait cycle, the double integration approach consists of three main steps (Hannik et al., 2018):
- Estimation of sensor orientation from the gyroscope data and transformation of the data into a global coordinate system.
- Gravity cancellation and double integration of the accelerometer data.
- Correction of the integration results by observing different boundary conditions
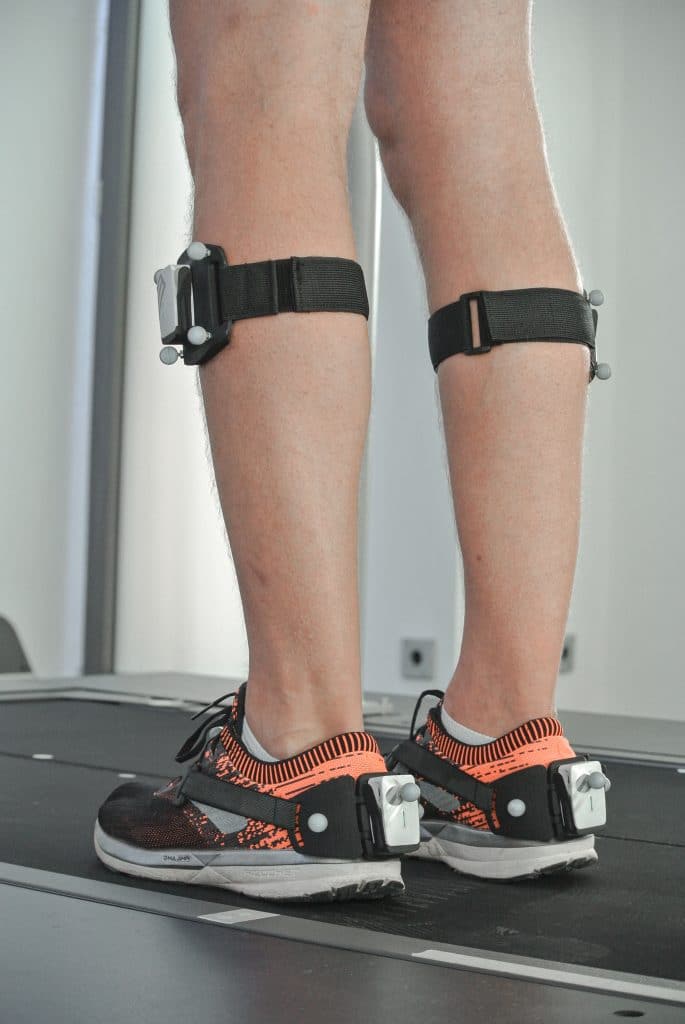
Challenges of estimating step length
Hannink describes in his 2018 publication that despite the progress in the field of step length estimation, the achieved measurement accuracy using IMUs is not sufficient for many medical issues at the current time. Three years later, developing algorithms with high accuracy in step length estimation using inertial sensors is still an open challenge (Mileti et al, 2020). The importance of high accuracy in step length estimation is necessary in a clinical context and can be seen in the following examples.
Hass et al. (2012) investigated mean step length in Parkinson’s disease (PD) patients with progressive disease. Between PD patients with minimal impairment and PD patients with very strong motor impairment, a reduction in step length of 11 cm was found (Hass et al., 2012). In this case, the precision of step length estimation should be at least 5 cm to detect changes (Hannink et al., 2018).
The importance of high precision becomes even more apparent when considering the decrease in step length with age in healthy subjects. Hollman et al. (2011) compared step lengths between the age groups 70-75 years and 85+ years. The study found an annual decrease in step length of 1 to 2 cm (Hollman et al., 2011). To determine this decrease, step length would need to be estimated with an accuracy of 5 mm (Hannink et al., 2018).
But what makes step length determination so complex?
Human gait is extremely complex and represents an interaction of the extremities, muscles, and tendons. In addition, the three main joints of the lower body and pelvis work together. During a gait cycle, the body moves not only from left to right but also up and down. This complexity means that biomechanical models can never capture reality in its entirety, but simplifications and assumptions must be made. In addition, anthropometric data are required for modeling, the acquisition of which is time-consuming and error-prone.
The double-integration approach as an alternative also has some challenges. The biggest is minimizing the integration drift, which is caused by the measurement error of the inertial sensors and increases to infinity in time. A common method of correction is to find points in time when the foot is not moving, meaning its velocity is zero. However, this correction is insufficient for a large number of gait disorders due to an altered gait pattern. An example of this is the gait pattern of patients with “pes equinus”, a disorder where the heel does not touch the ground during gait. As a result, there is no time when the foot is at rest.
The future outlook
This article has presented, on the one hand, the importance of step length in the clinical context, but also the dangers of insufficient estimation. New solutions are needed in order to overcome the challenges and make IMU technology even more valuable in clinical gait analysis.
The use of artificial intelligence (AI) represents a great potential. Especially the use for pathological data is promising, because in contrast to the previously mentioned methods neither a modeling of the gait pattern nor a correction is necessary. As a subfield of AI, it is possible to extract features from inertial sensor data using machine learning algorithms, as well as to detect patterns in the data that have remained hidden using classical approaches (Halijaj et al., 2018).
Author
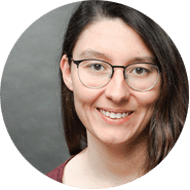
Laura Steffny
Biomechanical Engineer at Motesque